In the pursuit of materials with properties that don’t exist in nature, UC Santa Cruz researchers devised a new method for materials engineering that incorporates novel mathematical and deep learning techniques. This unique approach earned the researchers, including Ph.D. student Iman Nodozi in the Electrical and Computer Engineering department and his advisor Applied Mathematics faculty Abhishek Halder, the prestigious 2024 O. Hugo Schuck Best Application Paper Award from the American Automatic Control Council.
The paper that details this research was the result of collaboration between Nodozi and Halder with Jared O’Leary and Ali Mesbah at UC Berkeley. The public award ceremony for their awards took place this week at the 2024 American Control Conference in Toronto, Canada.
Material design
Material engineers work to create new materials with properties or structures that don’t naturally exist. This requires a forcing of some kind — applying heat, electromagnetic energy, or other properties to make the material behave how the engineers want.
“The idea is that you want to engineer materials with certain desired properties which would be relevant to their target applications — this could be unusual magnetic properties, electrical properties, or other mechanical or optical properties that do not exist in nature,” Halder said.
Once engineers discover that it is possible to create a material with a certain set of properties, such as a specific crystal structure, it’s important to figure out how to create that property with the least amount of forcing, a technique called “minimum effort stochastic control.” This is not just a matter of pushing the technology — it translates directly to the expense of manufacturing the material.
“Minimum effort directly translates to cost — you want to avoid expense, and at the same time, guarantee reproducibility and robustness despite noisy molecular-level dynamics,” Halder said.
This research mathematically guarantees that the control algorithm designed to force the crystal structure uses as minimum effort as possible, among all the possible control algorithms.
Novel approach
In this paper, the researchers explored new methods to force a certain structure of colloids, a general term for particles suspended within a substance. They investigated how to best use an electric field to force the colloids from a random, disordered structure to a defined, ordered structure over a time period of 200 seconds. Their method was demonstrated on a known benchmark problem in the field.
To do so, the researchers took a feedback control approach, meaning the equation or algorithm for finding a solution incorporates feedback from the underlying complex molecular process. But because the researchers are working with materials for which the chemical and quantum physics are quite complicated — both nonlinear and noisy — the design of high performance control is very challenging.
A typical feedback control approach uses point-to-point control. Instead, the researchers took a novel distribution-to-distribution approach, with each distribution modeling a measure of the randomness of the structure of the material. This allowed them to better solve how to take the colloids from a disordered structure to a defined structure via electric force over exactly the prescribed 200 second deadline.
Using this approach, the researchers discovered that their process led them to the creation of a non-conventional set of coupled nonlinear partial differential mathematical equations which had never been studied before. This adds value to the research on the theoretical mathematics side, advancing the mathematics of a branch of control theory called stochastic control, in addition to the applications for materials engineering.
“It turns out this opens up a class of problems which, from a mathematical point of view, had not been studied or solved before,” Halder said. “From our point of view, we had been working on this kind of problem of controlling the probability distribution for some time. But this particular class of problem gives rise to a mathematical structure which has not been seen before, and where our previous algorithm or anyone else's existing algorithm cannot be applied.”
Leveraging AI
Because neither their own or any other researchers’ algorithms could help solve these mathematical structures that the team introduced, their next step was to come up with a new algorithm to try to solve this new set of mathematical equations.
To do so, Nodozi led the creation of an open-source physics-informed neural network to use as a computational tool to solve their new equations. Their proposed physics-informed neural network is different from those available in the literature, since conventional neural network architectures do not respect the distributional dynamics, and also are ill-defined for losses between distributions.
The output of the neural network is the optimal time-varying electric field to best force the colloidal assembly and create the desired ordered structure. The network is trained so that it is constrained to only produce a set of solutions that are physically possible considering the noisy, nonlinear chemical and quantum physics.
“The award we got is likely because we show that for this kind of application, we have a new type of problem which can be solved by a new type of neural network architecture,” Nodozi said. “It's an innovative, complicated new way to formulate and solve an existing problem.”
“There is a lot of interest in the intersection of AI and material design synthesis,” Halder said. “Traditionally, materials design has been a slow discipline with a lot of trial and error. AI methods are changing that, opening up promising directions from a technological point of view.”
Halder and Nodozi plan to further explore the applications of their new distribution-to-distribution approach in material engineering and beyond, and investigate fundamental mathematics for understanding and solving their new equations without a neural network.
This research was supported by a collaborative National Science Foundation grant "Learning and Distributional Feedback Control for Fabrication of Advanced Materials" led by Halder.
Researchers win best paper award for introducing new AI method for minimum-effort materials engineering
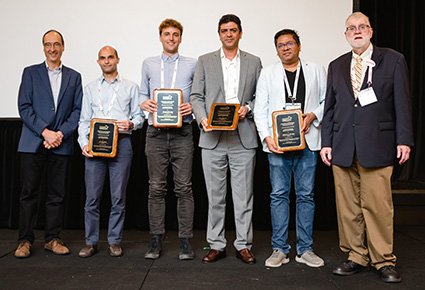